The Deutscher Wetterdienst (German Meteorological Service, DWD) provides operational seasonal climate predictions that are statistically downscaled for selected German cities. The new climate service, based on the German Climate Forecast System, was developed within the European Union Horizon 2020 project e-shape and is part of the DWD climate prediction website at www.dwd.de/climatepredictions.
SASKIA BUCHHOLZ, ANDREAS PAXIAN, BIRGIT MANNIG, AMELIE HOFF
Deutscher Wetterdienst
Potential impact of the service. The DWD recently launched a new climate service for selected German cities based on seasonal climate predictions for the two variables, near-surface (2 m) temperature and precipitation. The new service aims at decreasing the vulnerability of urban population to hazardous weather events and risks caused by climate variability. Climate predictions for urban areas can provide the scientific basis for midterm planning decisions. The new product is tailored for city authorities helping to plan and prepare, e.g., for periods of anomalous high temperatures, so that preventive measures can be taken in the occupational health and safety sectors. With regard to long-lasting dry periods, the decision makers can plan the distribution of water resources among different sectors (e.g., agriculture, industry, and urban green irrigation) more effectively.
Description of the pilot. Seasonal predictions give a prognosis on the development of temperature and precipitation for the coming 1–6 months. The German Climate Forecast System Version 2.1 (refined version based on GCFS2.0) (Fröhlich et al. 2021) provides the operational seasonal predictions for the new climate service. It is based on the global coupled earth system model of the Max Planck Institute for Meteorology (MPI-ESM-HR) (Müller et al. 2018, Mauritsen et al. 2018). Exact initial conditions of a forecast are crucial for the forecast skill. For the climate forecast to be as close as possible to the real state of the atmosphere, observations are assimilated into the MPI-ESM-HR prior to the start of the predictions. The current GCFS2.1 uses continuous nudging to bring the state of the model climate close to the observation-based state of the climate (Baehr et al. 2015, Fröhlich et al. 2021).
For a robust statistical estimate of the quality and reliability of the predictions, a large number of historical forecasts starting in the past (also called re-forecasts or hindcasts) are calculated for each forecast and compared to observations. Each forecast starts with slightly varying initial conditions of the climate system. The resulting variety of solutions, also called ensemble, is used to evaluate the uncertainties caused by uncertainties in the assimilated observations and the non-linearity of the climate system. In GCFS2.1 the ensemble is generated by different methods in atmosphere and ocean. For the hindcasts, GCFS2.1 starts 30 ensemble members for each calendar month in the years between 1990-2020. Real-time forecasts are performed with 50 ensemble members per forecast to achieve even more robust forecasts than the 30 members in the past (for which 50 members would not be feasible due to the high computational demand).
For Germany, the spatial resolution of the global climate predictions is increased using the empirical-statistical downscaling method EPISODES (Kreienkamp et al. 2019). EPISODES consists of two modules. In the first step, the statistical relationship between large-scale processes (e.g., large-scale relative humidity fields), prescribed by reanalysis data or the global climate forecast model and high-resolution precipitation and temperature observations, are defined by the combination of the selection of analogue days with a follow-up regression. In the second step, multivariate time series are created with a weather generator on the high-resolution target grid for various output variables. The HYRAS gridded data (Razafimaharo et al. 2020, Rauthe et al. 2013) are used as near-surface observational data for the downscaling. The EPISODES output has a horizontal grid point distance of about 5 km. The seasonal prediction for each city is an average of all grid boxes within the city boundary. In the case the city area is small, fewer than the usual minimum number of 9 grid boxes are used for aggregation. This is still feasible as the EPISODES output is no dynamical model output (which needs to be aggregated for larger areas) but is based on real observations.
The new service includes maps (Fig. 1), time series (Fig. 2) and tables of the ensemble mean and probabilistic predictions in combination with the prediction skill of temperature and precipitation for the 16 German state capital cities and Aschaffenburg (e-shape partner for the service development).
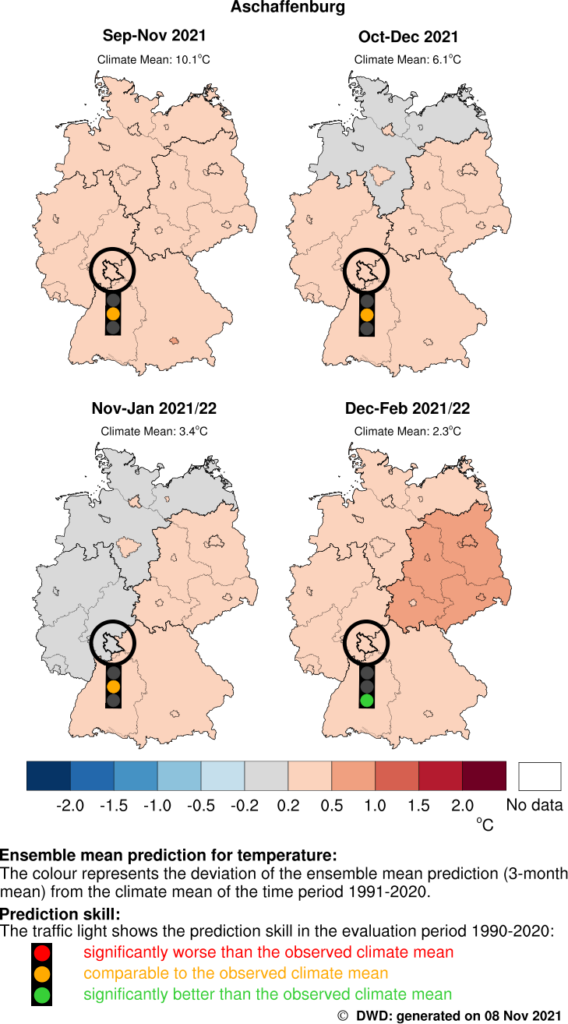
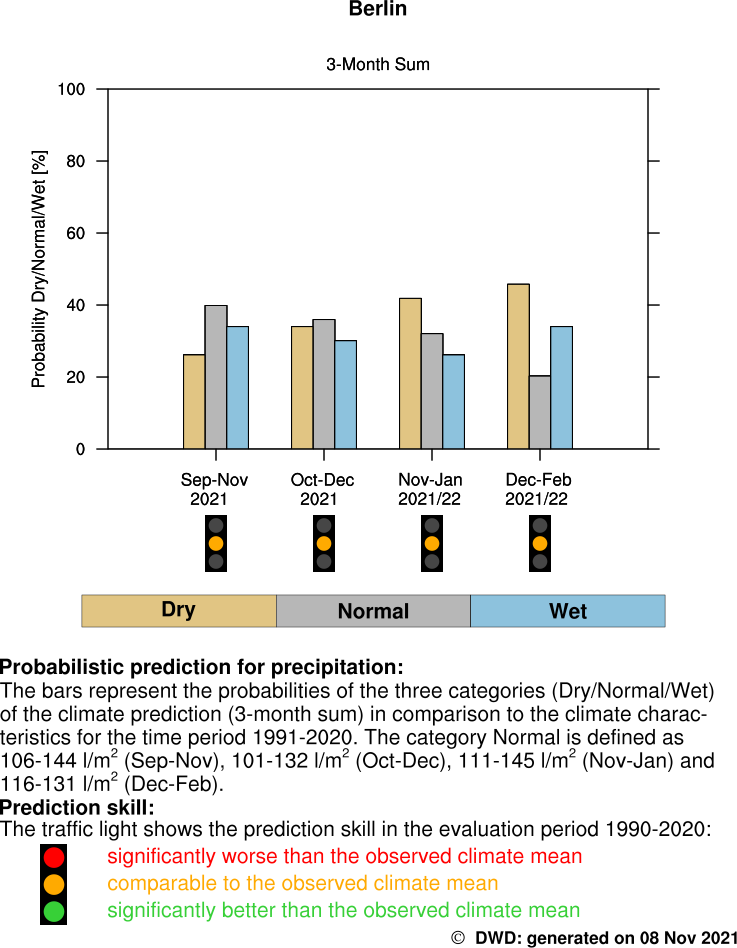
The quality (i.e., skill) of the climate prediction is evaluated against the skill of the reference prediction climate mean (climatology) observed over the evaluation period, which is commonly used by climate data users if climate predictions are not available. For the evaluation we use all available hindcasts, which encompass the initialisation years 1990–2020. The skill score of the ensemble mean prediction is determined using the skill score of the mean-squared error between the ensemble mean of the hindcasts and the actual observation (MSESS). The prediction skill score of the probabilistic predictions is determined using the ranked probability skill score (RPSS), which examines to which extent the predicted probabilities of occurrence of the categories (e.g., ‘below normal’, ‘normal’, ‘above normal’, thresholds are the 33rd and 66th terciles of the reference period 1991–2020) agree with the category actually observed. The reference prediction for the skill score is the observed climatology. A bootstrapping method is then applied to verify whether the skill comparison between hindcasts and a reference prediction is subject to accidental variations due to small sample sizes (significance test).EPISODES conserves the skill of the downscaled hindcasts for the two variables, near-surface (2 m) temperature and precipitation. Thus, the seasonal information is available at a higher spatial resolution without losing skill. Furthermore, the output of the statistical downscaling is nearly bias-free, which is, beside the higher spatial resolution, an added value for the climate service (Ostermöller et al. 2021).
Co-design. The design of the DWD climate prediction website has been developed in close cooperation with users from various sectors. At the beginning of the service development for German cities, a small survey was carried out among eleven cities for their requirements on the climate prediction products. During two individual meetings with representatives from the municipality of Aschaffenburg (departments of urban planning and environmental protection), the city’s individual needs for the service (e.g., graphs and description of temperature extremes and threshold exceedances, information on hot and dry episodes and heavy precipitation) could be discussed. During the last decade, city authorities in Germany have gained knowledge to deal with climate projections, impacts and uncertainties. Despite the achieved goals in the interpretation of climate projections, there are still knowledge gaps for the vast majority of cities when it comes to climate predictions and their added value.
The website content is presented in two sections, ”basic” and ”expert”, to cater for the specific needs of different users. The ”basic climate predictions” place a particular emphasis on comprehensibility, while the ”expert climate predictions” offer more detailed information, including prediction skill maps. City authorities attach great importance to data quality and uncertainties. To offer a low-threshold access to this information, a user-oriented traffic light shows at first glance the qualitative information about the prediction skill (”basic climate predictions”). It indicates whether regional mean climate predictions are significantly better (green), not significantly different (yellow) or significantly worse (red) than the reference prediction. A feedback formula on the website offers the possibility to express suggestions, praise, and criticism. Furthermore, the DWD holds an annual workshop for end-users and informs twice per year about new innovations and changes via a newsletter.
Acknowledgements: We thank Kristina Fröhlich, Sascha Brand and Katharina Isensee for their support with GCFS2.1, as well as Philip Lorenz and Frank Kreienkamp for their support and further adjustments of the EPISODES model. Besides, we would like to thank Klaus Pankatz for his help with the evaluation, Miriam Tivig for her support with the climate forecast website and Barbara Früh for her conceptual guidance. The e-shape project is funded under the European Union’s Horizon 2020 research and innovation programme under grant agreement 820852.
References
Baehr J., and Coauthors, 2015: The prediction of surface temperature in the new seasonal prediction system based on the MPI-ESM coupled climate model. Clim. Dyn., 44, 2723–2735, https://doi.org/10.1007/s00382-014-2399-7.
Fröhlich, K., and Coauthors, 2021: The German Climate Forecast System: GCFS. J. Adv. Model. Earth Syst., 13(2),https://doi.org/10.1029/2020MS002101.
Kreienkamp, F., A. Paxian, B. Früh, P. Lorenz, and C. Matulla, 2019: Evaluation of the empirical-statistical downscaling method EPISODES. Clim. Dyn., 52, 991-1026. https://doi.org/10.1007/s00382-018-4276-2.
Mauritsen, T., and Coauthors, 2018: Developments in the MPI-M earth system model version 1:2 (MPI-ESM1.2) and its response to increasing CO2. J. Adv. Model. Earth Syst., 11(4), 998-1038, https://doi.org/10.1029/2018MS001400.
Müller, W. A., and Coauthors, 2018: A Higher-resolution Version of the Max Planck Institute Earth System Model (MPI-ESM1.2-HR). J. Adv. Model. Earth Syst., 10(7), 1383-1413, https://doi.org/10.1029/2017MS001217.
Ostermöller, J., P. Lorenz, K. Fröhlich, F. Kreienkamp, and B. Früh, 2021: Downscaling and Evaluation of Seasonal Climate Data for the European Power Sector. Atmosphere, 12(3), 304, https://doi.org/10.3390/atmos12030304.
Rauthe, M., H. Steiner, U. Riediger, A. Mazurkiewicz, and A. Gratzki, 2013: A Central European precipitation climatology – Part I: Generation and validation of a high-resolution gridded daily data set (HYRAS). Meteorologische Zeitschrift, 22(3), 235-256, https://doi.org/10.1127/0941-2948/2013/0436.
Razafimaharo, C., S. Krähenmann, S. Höpp, M. Rauthe, and T. Deutschländer, 2020: New high-resolution gridded dataset of daily mean, minimum, and maximum temperature and relative humidity for Central Europe (HYRAS). Theor. Appl. Climatol., 142, 1531-1553, https://doi.org/10.1007/s00704-020-03388-w.
HOW TO CITE THIS ARTICLE:
Buchholz S., A. Paxian, B. Mannig, and A. Hoff, 2022: Seasonal climate predictions for German cities to strengthen urban resilience to climate variability, FMI’s Clim. Bull. Res. Lett., 4(1), 17–20, https://doi.org/10.35614/ISSN-2341-6408-IK-2022-06-RL.
CITATION INFORMATION:
Authors: Saskia Buchholz, Andreas Paxian, Birgit Mannig, Amelie Hoff
Received: November 26, 2021
Accepted: May 31, 2022
First online: June 02, 2022
Published: September 02, 2022
Journal: FMI’s Climate Bulletin Research Letters
Volume: 4
Issue: 1
Pages: 17–20
DOI: https://doi.org/10.35614/ISSN-2341-6408-IK-2022-06-RL
This article is part of the e-shape Special Issue.
Header image: Pixabay